AI and Edge Computing: Revolutionizing the Future of Fleet Telematics
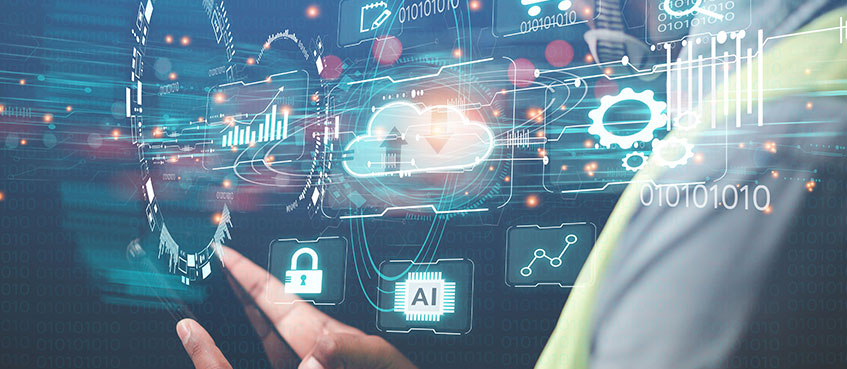
Introduction:
In the ever-evolving landscape of transportation and logistics, the integration of cutting-edge technologies has become instrumental in reshaping the efficiency and effectiveness of fleet management. Two key technologies leading this transformation are Artificial Intelligence (AI) and Edge Computing. As we delve into the synergy of AI and Edge Computing, it becomes evident that they are poised to revolutionize the future of fleet telematics.
Understanding Fleet Telematics:
Fleet telematics involves the use of technology to monitor, analyze, and manage a fleet of vehicles. This includes gathering data in real-time from various sensors and devices installed in vehicles, allowing for comprehensive insights into vehicle performance, driver behavior, and overall fleet operations.
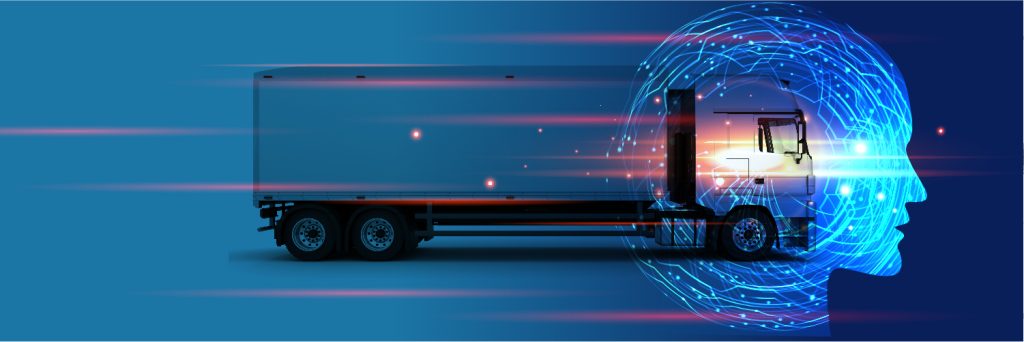
The Role of AI in Fleet Telematics:
Predictive Maintenance: AI algorithms analyze real-time data to predict when a vehicle is likely to require maintenance. This proactive approach minimizes downtime, reduces maintenance costs, and ensures that vehicles are in optimal condition.
Route Optimization: AI-powered route planning takes into account various factors such as traffic conditions, weather, and historical data. This results in more efficient routes, reducing fuel consumption, and improving delivery timelines.
Driver Behavior Monitoring: AI algorithms analyze driver behavior data, providing insights into factors like speed, braking patterns, and adherence to traffic rules. This not only enhances safety but also allows for targeted driver training programs.
The Role of Edge Computing in Fleet Telematics:
Real-time Data Processing: Edge computing involves processing data closer to the source (in this case, vehicles) rather than relying solely on centralized cloud servers. This enables real-time data processing, reducing latency and enhancing responsiveness.
Bandwidth Efficiency: By processing data at the edge, only relevant information is sent to the cloud, reducing the need for large data transfers. This not only saves bandwidth but also ensures that critical decisions can be made on-site without relying on constant connectivity.
Enhanced Security: Edge computing adds an additional layer of security by processing sensitive data within the local environment. This minimizes the risk of data breaches during data transfer between vehicles and central servers.
Benefits for Fleet Management:
Cost Efficiency: The combination of AI and Edge Computing leads to cost savings through optimized routes, reduced maintenance expenses, and improved fuel efficiency.
Operational Efficiency: Real-time data processing at the edge enhances overall operational efficiency by providing instant insights for better decision-making.
Scalability and Flexibility: AI and Edge Computing solutions are scalable, allowing fleet managers to adapt and expand their systems as their operations grow. This flexibility ensures that technology evolves with the fleet's changing needs.
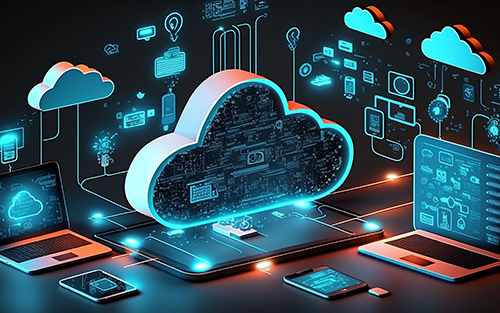
Conclusion:
As we stand at the intersection of AI and Edge Computing, the future of fleet telematics looks promising. The seamless integration of these technologies empowers fleet managers with unprecedented levels of data-driven insights, operational efficiency, and cost savings. Embracing the transformative potential of AI and Edge Computing is not just a leap into the future; it's a strategic move toward a more connected, intelligent, and efficient fleet management ecosystem. The road ahead is paved with innovation, and the marriage of AI and Edge Computing is steering the fleet telematics industry toward a future of unparalleled advancements.